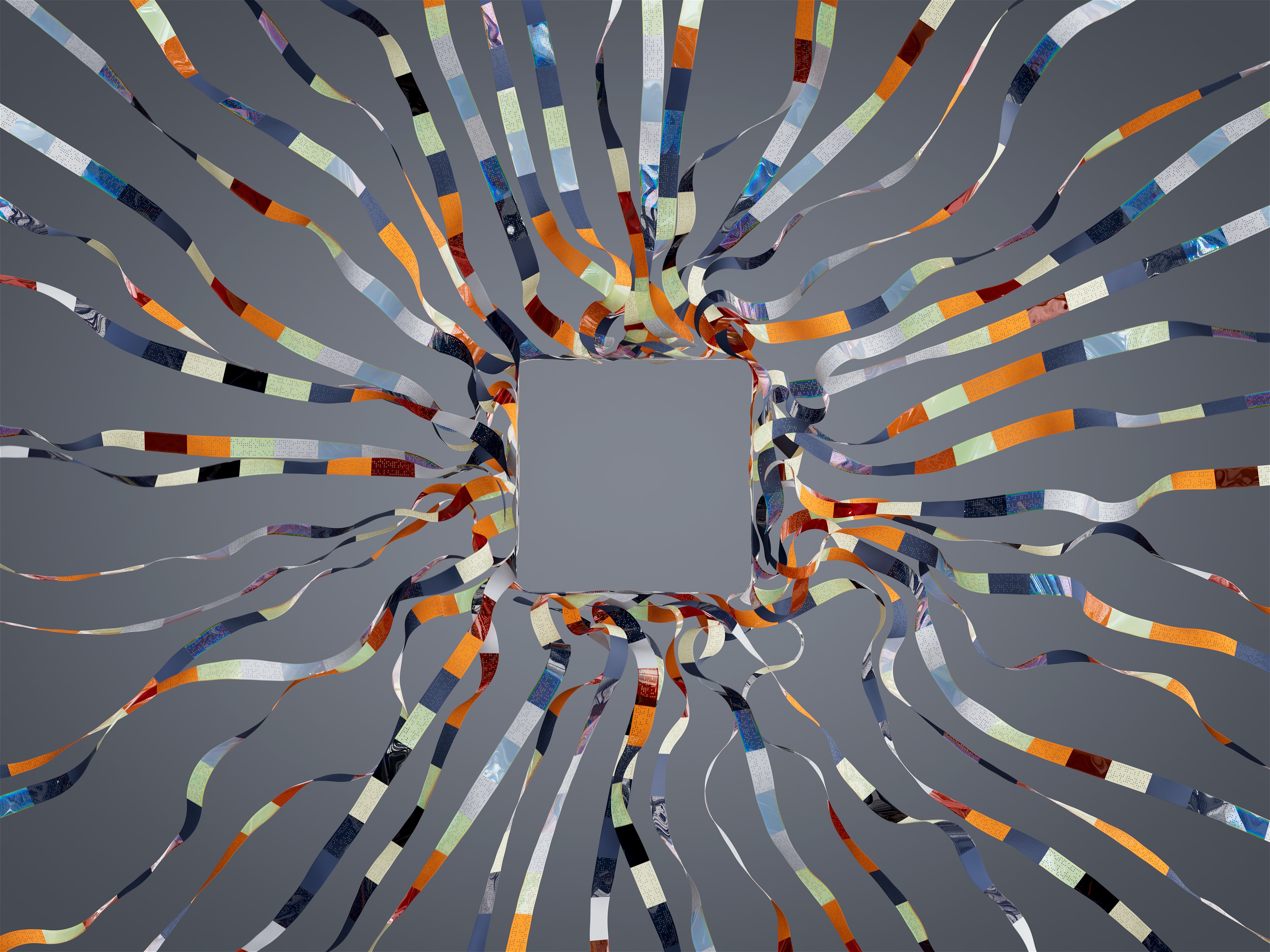
Perspectives
From Risk to Reward: The Impact of ChatGPT and LLMs on Underwriting
Insurers are trying to figure out how to leverage the newest advances in AI, so we compiled the go-to guide.
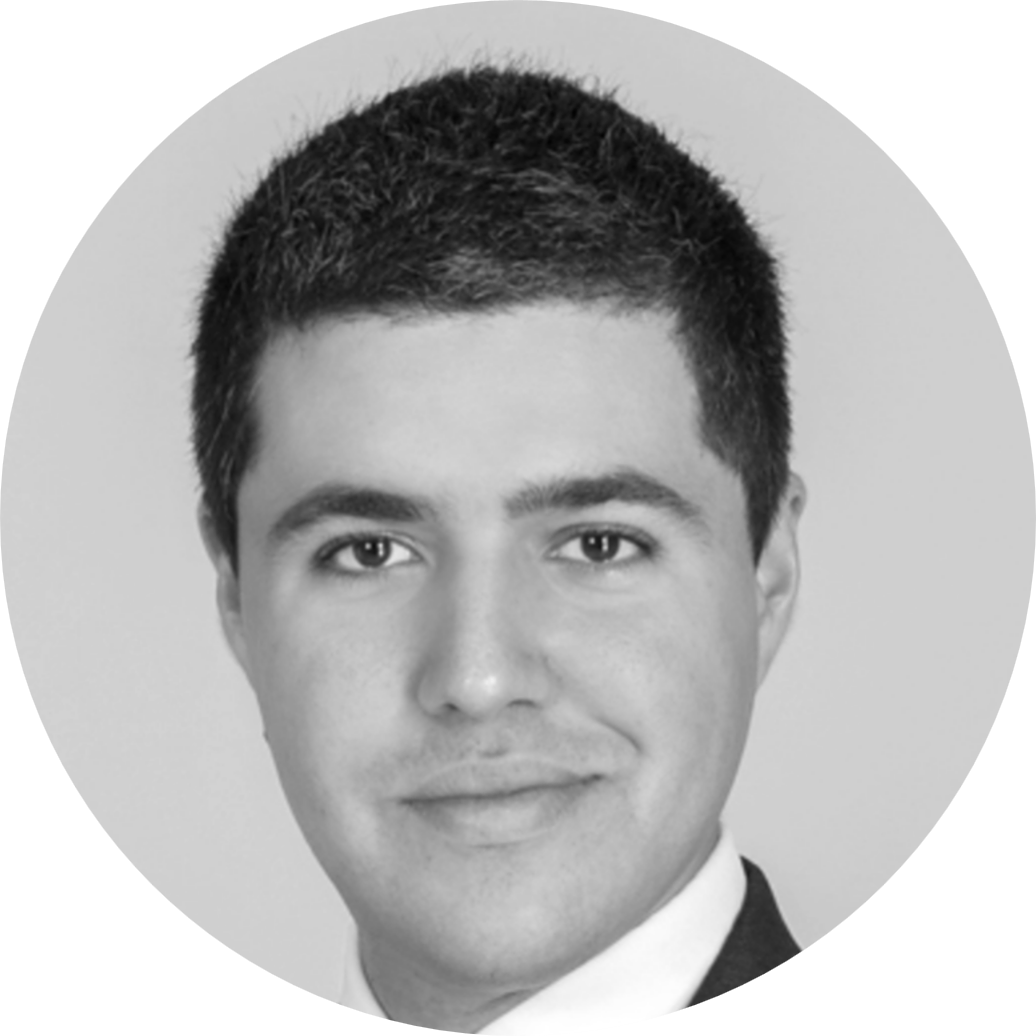
Paul Monasterio
9 mins
CPCU, ARM, AU...AI?
Artificial Intelligence is everywhere these days. Ever since OpenAI launched their ChatGPT product – which set records with 100 million users in its first two months – AI and LLMs (large language models) have taken over cocktail conversations and board meeting agendas.
But first, if you haven’t seen LLMs yet, here is one of the many crazy videos of what they can do, courtesy of Google:
Each new day sees the launch of dozens of brand-new products built around AI, each one of them transforming an industry or our personal lives.
This has left many underwriters and underwriting leaders wondering: “What does all of this mean for me?”
How have insurers used AI in the past?
First, let’s take a step back to recognize that data science and analytics aren’t new to insurance.
Models, data, and insurance have always been intertwined. In fact, many people consider actuaries to be the first data scientists – their statistical risk models determined pricing for countless policies hundreds of years before calculators came along. Over time, as technology and algorithms evolved, so did insurers.
In fact, carriers (and later MGAs/MGUs) have already implemented various approaches to AI over the years:
- Rules-based models – The simplest form of artificial “intelligence”, rules-based models follow the logic of “If this is true, then do this”. The first steps of insurance automation revolved around rules, from underwriting guidelines to claims-handling tasks.
- Predictive analytics – With enough historical data on characteristics and outcomes, insurers could begin building models that predicted the likelihood or magnitude of an outcome, like catastrophe models, with increasing accuracy.
- RPA (Robotic Processing Automation) – RPA allowed carriers to fully automate rules-based rote tasks, freeing up their employees to focus on items that required intelligent decision-making.
- AI / ML (Artificial Intelligence / Machine Learning) – These models, which “learn” from high volumes of historical data, can predict actual values or classify items with tremendous accuracy. Insurers have used ML models for everything from automating inspections with a smartphone to predicting underwriting fraud.
- GenAI / GPT / LLMs (Generative AI / Generative Pre-trained Transformer / Large Language Models) – These models include ChatGPT and Google Bard. LLMs have exploded into the public consciousness over the last six months and are trained on massive amounts of data – think a significant percentage of the Internet – using truly awesome computational power. These models can interpret full sentence requests or even images (with the appropriate context) and generate (hence the name) sensible, informed responses in human-like text.
With LLMs being so new - and with the challenges that come with that newness, like “hallucinations,” misinformation, security, and complicated prompt engineering - the vast majority of insurers have not yet adopted them into their workflows. So what do underwriting leaders need to know about LLMs to get the most out of them? To start, they need to know where to use them.
Using GenAI and LLMs for Automation vs. Augmentation
Throughout all of these evolutions in AI, technology enhancements for underwriters have generally fallen into one of two categories:
- Automation - Taking work out of underwriters’ hands, and
- Augmentation - Accelerating, improving, and organizing underwriters’ work
Both of these categories are vital for modernizing insurance operations and hitting targets. However, it’s critical to use the right algorithms in the right situations.
Here’s a prediction - the insurers who reap the biggest benefits from LLMs in the next five years will be those who adopt effective augmentation strategies, not automation strategies.
Why?
While large language models (LLMs) are excellent at many augmentation tasks, like summarizing lengthy documents or generating high caliber writing, there is one key area they struggle: judgment. We’ve already seen horror stories about how poor implementation of these models can lead to disaster. Plus, ChatGPT is notorious for being overly confident in every answer and making up an answer when it’s not sure – it’s not built to say “I don’t know.”
As a result, these models are simply not capable of reliably making underwriting decisions without human involvement – and they’re not close. On top of that, insurers should be concerned about black box models, potential bias, security risks, and associated regulatory obstacles.
All of this means that underwriters will continue to be the cornerstone of commercial underwriting. And since underwriters aren’t going anywhere, finding ways to augment and enhance their day-to-day decisions is critical when implementing GPT models. All told, we are confident - and have been since day 1 at Kalepa - that the future of underwriting will be driven by the companies who can combine the best of human intelligence with the best of artificial intelligence.
How Can GenAI and LLMs Help Insurers Right Now
So, if underwriter augmentation is the key for taking advantage of LLMs, what can carriers and MGAs/MGUS do today to improve their underwriting? Several parts of the underwriting life cycle are ripe for transformation – if insurers can implement these algorithms effectively.
Submission Ingestion
Like it or not, submissions aren’t getting any cleaner. Thankfully, when trained appropriately, LLMs are adept at taking complex, unstructured text and making it easy to understand. For example, LLMs can extract key insights from broker and agent emails, even performing accurate business classification from just a few sentences.
When combined with computer vision algorithms like optical character recognition (OCR), LLMs can identify critical facts from forms like loss runs, supplemental applications, ACORD forms, and financial statements. This saves underwriters a tremendous amount of time – after all, per Accenture, 40% of underwriters’ time is spent on administrative tasks.
Research and Analysis
LLMs are excellent at processing, summarizing, and analyzing blocks of text. You probably already know this, but the vast majority of underwriting information is stored in blocks of text. For example:
- News articles – There could be dozens or hundreds of news articles about an insured – particularly those with large multi-location submissions.
- Websites – LLMs can scan through an insured’s website to identify crucial facts about business operations – all in seconds.
- Legal Filings – Legal filings are notoriously lengthy and hard to digest, but LLMs can easily summarize them in an underwriting-specific context for rapid review.
- Online Reviews – Even with reviews spread across many platforms and written with varying quality, LLMs can find hidden exposures like infestations in a hotel or shoddy workmanship and construction defects performed by a general contractor.
Drafting Narratives and Communications
Despite decades of technological evolution, commercial insurance underwriting is still built on a foundation of relationships. High performing underwriters are expected to provide excellent customer support to their distribution partners, and building great relationships with brokers and agents is a critical part of the job. So while automating all correspondence with distribution partners is out of the question, LLMs can free up underwriters to spend more time on the relationships that matter.
LLMs can prepare draft emails for underwriters based on a few quick prompts. Of course, underwriters will want to add tweaks before sending, but this can help underwriters get their messages out quickly while maintaining that personal touch.
LLMs can also help compile internal documentation on each account. By running on underwriters’ file notes and all of the ingested submission info, these models can compile a summary of the account and the underwriting determinations.
Challenges with Using LLMs for Underwriting Today
We’ve highlighted several ways you can already start using LLMs like ChatGPT to improve your underwriting. However, getting started isn’t as easy as you might think. Here are a few pitfalls to watch out for before jumping in headfirst.
Security
Information security is paramount for information generated by the insurer or provided by the insured – this is especially true for personally identifiable information (PII).
Do you know what happens with the data you enter into ChatGPT?
It actually stores all of the information without any security, encryption, or privacy considerations.
There are ways to use GPT more securely, but they also take more work. For one, it’s possible to set up private GPT instances that keep data access restricted to a given organization. This involves a decent amount of IT work and API setup, but it is feasible for sophisticated IT teams. Also, by building a secondary model to pull out and consolidate non-sensitive information, smart tech teams can set up automated workflows that leverage LLMs for the same tasks without exposing confidential details.
Prompt Engineering
While it’s easy to get okay results from ChatGPT, getting consistent, specific, accurate, and relevant results requires prompt engineering - basically being good at asking questions to the model. The better you are, the better the outputs will be. Already, companies are hiring dedicated prompt engineers who specialize in getting the maximum return from every GPT call.
How much does prompt engineering really matter? Here’s the difference between basic prompting and higher-quality prompting in ChatGPT:
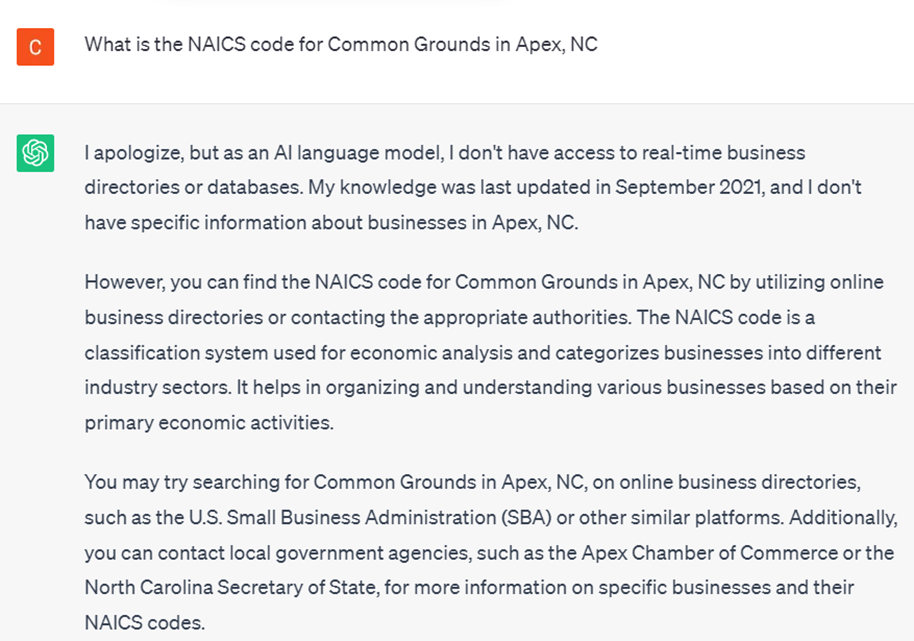
Compare that to:
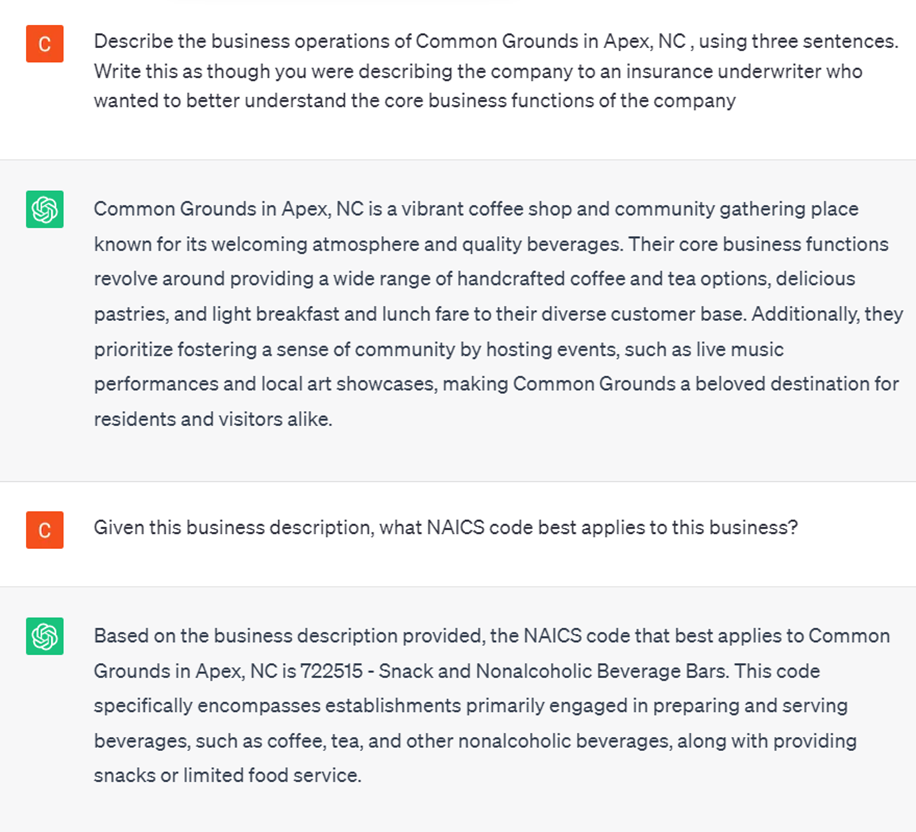
This is only a simple example - if you want to efficiently augment your underwriters’ work (or automate simple tasks) with LLMs, high quality prompt engineering is a must.
Given ChatGPT’s tendency to “hallucinate” answers, there are many edge cases to handle and lots of testing is required before moving anything to production. When using third party LLMs, ongoing model monitoring is also critical, since the underlying large language model is constantly changing - and outside of prompt engineering, it’s completely out of your control.
Implementation
Like with any new technology, the biggest challenge for insurance companies is often implementation. That includes technical evaluation, security reviews, procurement processes, personnel allocation, integration, testing, and change management. All these hurdles can stall out innovation. According to BCG, only 30% of IT transformations are actually successful.
In order to integrate LLMs into their underwriting workflow, insurers need to connect the models with:
- The submissions
- External data
- The interface for the underwriters
The reality is that most insurance companies still have not integrated those three. Submissions live in raw emails in Microsoft Outlook, external data lives in separate portals with unique logins and lookups, and the underwriting workbench is antiquated, ignored, or nonexistent. But without connections between these three pillars, LLMs have zero opportunity to provide value.
Given how fast the world of AI is evolving, by the time many insurers integrate these three AND implement LLMs, the Next Big Thing will already be out – and the insurer will be missing out.
In order to succeed, carriers and MGAs/MGUs need to determine how they can accelerate LLM implementation. For all but the most technically sophisticated of carriers, this will likely include partnering with a software company that has already completed most of the leg work.
AI Insurtechs and other software companies are already handling many of these security and prompt engineering challenges, and they have already developed an expertise in how to implement their solution. Buyer beware, there is definitely a spectrum here – many of these companies also have slow implementation timeframes or aren’t leveraging LLMs very well – so be sure to do your research.
How is Kalepa Using LLMs?
When we founded Kalepa over five years ago, we did so because we felt that commercial underwriters could be empowered by better uses of artificial intelligence. With every year since, that’s become increasingly true.
Over the past several months, we’ve deployed GPT-powered enhancements to our Copilot underwriting workbench. Copilot also tackles the three implementation pillars out of the box, by automating submission ingestion, sourcing and centralizing third party data, and displaying everything in an interface that helps underwriters write complex risks 58% faster. We've also taken all the necessary precautions to ensure that everything we do with our clients' data adheres to critical security and privacy standards. That means Kalepa’s clients get all of the benefits of LLMs without any of the legwork.
Our clients are already taking advantage of these extra features - at no extra cost - by writing risks better and faster than ever.
News and Legal Filings Summaries
From the beginning, Copilot has been identifying exposures and controls for underwriters by crunching through millions of news articles and legal documents, identifying which ones are relevant to a business, and ranking them by their relevance to the coverage in question.
Now, Copilot delivers LLM-powered summaries of news articles and legal filings, so underwriters can get the recap without doing the reading. Copilot identifies the stories and filings that are actually relevant for underwriting and then extracts the key insights for rapid review. This helps underwriters quickly identify hidden exposures that impact quoting and rating decisions.
Advanced Data Extraction from All Submission Documents
We also supercharged the entire document extraction and processing pipeline.
What does that mean for underwriters?
- Highly detailed descriptions of operations for every business
- Automatic extraction of key exposure info from documents like project budgets
- Advanced ingestion of complex documents like supplemental applications and loss runs
Business Classification
We also leveled up our business classification models to provide even more accurate NAICS classification. This is especially true for construction and manufacturing submissions, where many legacy models fail.
How did we do it? We combined our proprietary prompt layer with tailor-made models from our database of over 30M businesses to provide classifications that go far beyond a simple Google search.
This gives our insurer partners confidence that every one of their industry-based underwriting guidelines will apply correctly, and they can ensure that every risk they bind is in appetite.
Upcoming Copilot LLM Features
Our data science team is hard at work developing additional features that automatically will roll out to our customers free of charge in the upcoming weeks:
- Drafting Correspondence – Coming soon, Copilot will augment underwriters’ conversations with distribution partners by drafting responses and questions. This will save underwriters time while maintaining the personal touch that excellent underwriters are known for
- Copilot Chat – Ask Copilot any question and it will give you an answer. Make it easier to find submissions that match anything you want, or answer a specific question about the details of a particular risk
- Drafting Narratives – Summarizing the details of every submission is a painstaking but important process. With this new feature, Copilot will provide draft narratives for underwriters so they can edit for details instead of writing from scratch
Integrating LLMs and GenAI is hard work. There are tremendous benefits to the carriers who can implement them effectively, but lots of pain lies ahead for those who can’t. Thankfully, here at Kalepa, we’ve done the hard work so you don’t have to.
Want to learn how Copilot can bring LLM capabilities to your team from day 1? Set up a demo and we’ll show you on your own submissions.
Want to get in touch?
Sign up for our newsletter for the latest Copilot news,
features, and events, straight from the source
Hassle-free setup
Hands-on training & support
See the value on day one